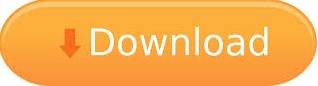

Mixed model designs are an extension of the general linear model, as in analysis of variance (ANOVA) designs. Each level is (potentially) a source of unexplained variability. Multilevel Analysis using the hierarchical linear model : random coefficient regression analysis for data with several nested levels. For example, the first-order model in two independent variables, Y = β0 + β1x1 + β2x2 + ϵ, is nested within the complete second-order model.

Two regression models are called nested if one contains all the predictors of the other, and some additional predictors. "lmerTest Package: Tests in Linear Mixed Effects Models." Journal of Statistical Software, 82(13), pp. To cite lmerTest in publications use: Kuznetsova A., Brockhoff P.B. This second argument, data, is optional but recommended and is usually the name of an R data frame. Like most model-fitting functions in R, lmer takes as its first two arguments a formula spec- ifying the model and the data with which to evaluate the formula. Use the Kenward-Roger approximation to get approximate degrees of freedom and the t-distribution to get p-values, which is implemented in the pbkrtest package.

Use the Satterthwaite approximation, which is implemented in the lmerTest package. Three ways to get parameter-specific p-values from lmerUse the normal approximation. This model assumes that slopes are fixed (the same across different contexts). What is a random intercept model?Ī random intercepts model is a model in which intercepts are allowed to vary, and therefore, the scores on the dependent variable for each individual observation are predicted by the intercept that varies across groups. So that means that the relationship between x1 and y is different for every group. Well, unlike a random intercept model, a random slope model allows each group line to have a different slope and that means that the random slope model allows the explanatory variable to have a different effect for each group. To do this, you compare the log-likelihoods of models with and without the appropriate random effect - if removing the random effect causes a large enough drop in log-likelihood then one can say the effect is statistically significant. How do you know if a random effect is significant? Statistical models always describe variation in observed variables in terms of systematic and unsystematic components. Random-effects models are statistical models in which some of the parameters (effects) that define systematic components of the model exhibit some form of random variation.
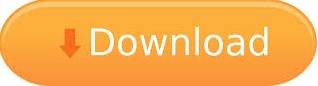